Notice
Recent Posts
Recent Comments
Link
일 | 월 | 화 | 수 | 목 | 금 | 토 |
---|---|---|---|---|---|---|
1 | ||||||
2 | 3 | 4 | 5 | 6 | 7 | 8 |
9 | 10 | 11 | 12 | 13 | 14 | 15 |
16 | 17 | 18 | 19 | 20 | 21 | 22 |
23 | 24 | 25 | 26 | 27 | 28 |
Tags
- Faster R-CNN
- R-CNN
- Region proposal Network
- object queries
- Map
- RoI pooling
- pytorch
- BiFPN
- multi task loss
- Fast R-CNN
- hard negative mining
- YOLO
- herbwood
- AP
- RPN
- fine tune AlexNet
- Average Precision
- Anchor box
- IOU
- Object Detection metric
- Darknet
- Non maximum suppression
- Linear SVM
- detr
- Detection Transformer
- Hungarian algorithm
- Multi-task loss
- Bounding box regressor
- Object Detection
- mean Average Precision
Archives
- Today
- Total
목록multi-scale (1)
약초의 숲으로 놀러오세요
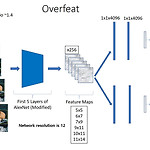
이번 포스팅에서는 Overfeat(Integrated Recognition, Localization and Detectionusing Convolutional Networks) 논문을 읽고 정리해봤습니다. 이전에 읽었던 R-CNN 논문에서 Overfeat보다 R-CNN이 더 좋은 성능을 보였다는 언급이 있어, 굳이 Overfeat을 공부해야할까 고민했지만, 논문을 직접 읽고 생각이 달라졌습니다. 논문에서 다른 detection 모델과는 다른 구조나 학습 방법 등 새로운 시도가 있어 흥미로웠습니다. 실제로 이러한 접근 방법은 One-stage detector의 시초로서의 면모를 보이며, SSD, YOLO 등 후속 모델에 큰 영향을 주었다고 합니다. Overfeat을 공부하면서 cogneethi님의 블로그와..
Computer Vision/Object Detection
2020. 12. 4. 20:12