Notice
Recent Posts
Recent Comments
Link
일 | 월 | 화 | 수 | 목 | 금 | 토 |
---|---|---|---|---|---|---|
1 | ||||||
2 | 3 | 4 | 5 | 6 | 7 | 8 |
9 | 10 | 11 | 12 | 13 | 14 | 15 |
16 | 17 | 18 | 19 | 20 | 21 | 22 |
23 | 24 | 25 | 26 | 27 | 28 |
Tags
- Map
- RPN
- RoI pooling
- Detection Transformer
- object queries
- Non maximum suppression
- Darknet
- pytorch
- Region proposal Network
- multi task loss
- BiFPN
- Object Detection metric
- Linear SVM
- IOU
- R-CNN
- fine tune AlexNet
- Bounding box regressor
- Anchor box
- YOLO
- Object Detection
- AP
- herbwood
- Faster R-CNN
- hard negative mining
- Hungarian algorithm
- Fast R-CNN
- Multi-task loss
- mean Average Precision
- Average Precision
- detr
Archives
- Today
- Total
목록object queries (1)
약초의 숲으로 놀러오세요
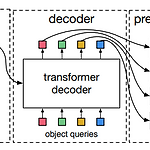
이번에는 ECCV 2020년에 발표된 DETR 논문(End-to-End Object Detection with Transformers)을 읽고 리뷰해도록 하겠습니다. DETR은 Transformer 구조를 활용하여, end-to-end로 object detection을 수행하면서도 높은 성능을 보였습니다. 현재 많은 SOTA 모델들이 DETR을 기반으로 발전한만큼, 반드시 읽어야하는 기념비적인 논문이라고 할 수 있습니다. Research gap 본 논문에서는 object detection을 bounding box와 category라는 set $G = \{(B_0, C_0), (B_1, C_1), …, (B_n, C_n)\}$을 예측하는 task로 정의합니다. 이 때 기존의 object detection ..
Computer Vision/Object Detection
2023. 1. 6. 17:08