Notice
Recent Posts
Recent Comments
Link
일 | 월 | 화 | 수 | 목 | 금 | 토 |
---|---|---|---|---|---|---|
1 | 2 | 3 | 4 | |||
5 | 6 | 7 | 8 | 9 | 10 | 11 |
12 | 13 | 14 | 15 | 16 | 17 | 18 |
19 | 20 | 21 | 22 | 23 | 24 | 25 |
26 | 27 | 28 | 29 | 30 | 31 |
Tags
- Hungarian algorithm
- AP
- Map
- Darknet
- pytorch
- hard negative mining
- detr
- Average Precision
- Anchor box
- IOU
- BiFPN
- Object Detection metric
- Linear SVM
- herbwood
- Non maximum suppression
- fine tune AlexNet
- Object Detection
- object queries
- Faster R-CNN
- RPN
- YOLO
- multi task loss
- Fast R-CNN
- mean Average Precision
- RoI pooling
- Multi-task loss
- Bounding box regressor
- Detection Transformer
- Region proposal Network
- R-CNN
Archives
- Today
- Total
목록False Positive (1)
약초의 숲으로 놀러오세요
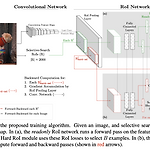
이번 포스팅에서는 OHEM(Online Hard Example Mining) 논문(Training Region-based Object Detectors with Online Hard Example Mining)을 리뷰해보도록 하겠습니다. 일반적으로 object detection 시, 배경 영역에 해당하는 region proposals 수가 더 많아 클래스 불균형(class imbalance)가 발생하고, 이러한 문제를 해결하기 위해 Hard Negative Mining 방법을 적용했습니다. 하지만 이로 인해 학습 속도가 느려지고, 성능 향상에 한계가 생기게 됩니다. 이러한 문제를 해결하여 모델의 학습 속도 개선과 성능 향상을 이뤄낸 새로운 bootstrapping 방법인 OHEM을 살펴보도록 하겠습니다...
Computer Vision/Object Detection
2020. 12. 24. 18:33